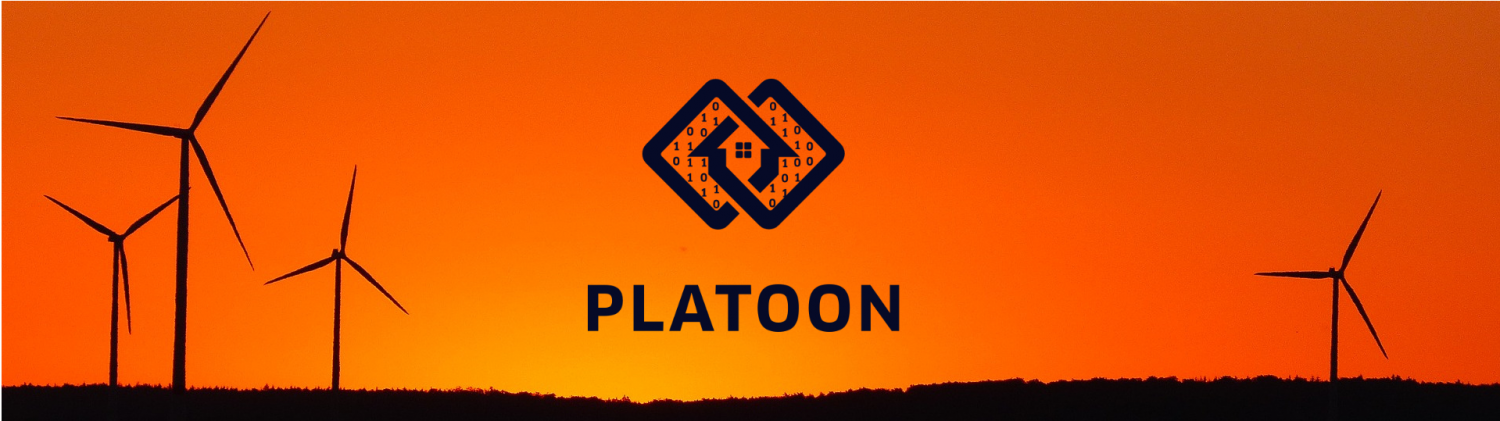
Pilot/Data Owner Objective:
Real Time Predictive Maintenance of Wind farms is an innovation trending topic because it is beneficial to many stakeholders at different positions in the value chain:
- The turbine owner will benefit from avoiding lost revenue from long downtimes due to unpredicted and thus unanticipated failures.
- The electricity grid TSO will benefit from a more stable grid because unexpected downtimes and thus large changes in available production capability in the electricity grid are avoided.
- The manufacturer of the wind turbine and power trains is interested in the knowledge of the real (and extreme) operation conditions the equipment is exposed to during operation.
The end goal of the pilot is to detect critical failures 50% faster than current techniques. This offers the time to the turbine owner to order spare parts, organise the maintenance action while keeping the turbine online.
Services offered by the PLATOON reference architecture’s components:
PLATOON will provide a set of data-analytics based services through the data analytics toolbox for continuously assessing the health of the drivetrain components of all the turbines in a fleet. Data from 110 Wind Turbines are accessible. Apart from that, the use of a digital twin approach combining physical models and machine learning models in a data-streaming context will help to foresee potential damages. The innovations in this pilot focus on:
- Automated anomaly detection in multi-stream datasets of different velocity
- Physics based digital twins to automatically annotate multi-stream sensor data to create optimized training datasets for machine learning models for system health degradation prediction.
- Integrated digital twins combining physics-based and machine learning models to predict failure based on anomalies and is able to learn the links between potential causes and the experienced failure. As such it will bring deeper understanding of the experienced failures and allow failure avoidance through design changes.